Artificial intelligence is at the heart of our society’s concerns today: platform recommendation systems, autonomous driving, and conversational generative AI such as ChatGPT, Mistral, Gemini and, more recently, DeepSeek.
These AIs are often referred to as ‘black boxes’, because the way they operate makes it difficult to understand the ‘path’ they have taken to make a decision or a prediction.
This behaviour is at the root of the notion of ethical AI.
Why ‘ethical’? Because it is important to ensure the safety and integrity of users by informing them of the data used to train these models.
In addition, it is essential to be able to ‘open the black box’ and make their inference (the result of their prediction) explainable.
Explainable artificial intelligence
eXplainable AI is an approach that makes it possible to understand the results and conclusions of machine learning algorithms, and to characterise their accuracy and potential biases.
The aim of all this is to control the data at the source of the model in order to boost user confidence, extend its uses and enable AI to be used more widely.
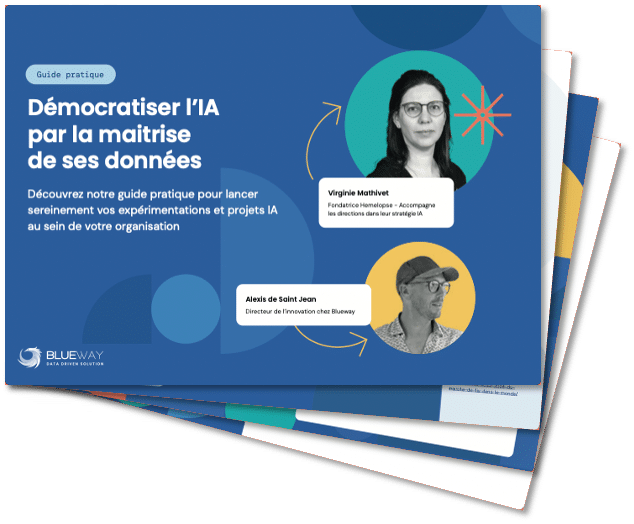
Démocratiser l’Intelligence Artificielle par la maîtrise de ses données
The advantages of AI explainability are notable:
- Increased reliability: By explaining the characteristics and rationale of the AI output, humans are more likely to trust the AI model, increasing the reliability of the AI.
- Regulatory compliance: In sectors such as finance and healthcare, integrating machine learning models into complex risk assessment strategies may be necessary to comply with regulatory risk management requirements.
- Ethical justification and bias removal: Because explainable AI is transparent and more easily debugged, unconscious bias can be avoided and ethical decisions can be explained.
- Usable and robust information: AI and machine learning offer the potential for actionable and robust information, but explainable AI allows humans to understand how and why the algorithm has made what it believes to be the best decision.
Explainable AI is even an obligation in certain areas where confidence in its results is fundamental. These areas are, for example:
- Healthcare: to establish diagnoses with complete transparency by tracing the decision-making process for patient care.
- Financial services: by promoting the reliability of their use in loan or credit approval.
- Justice and police investigations: by speeding up DNA analysis decisions and understanding the evidence behind them.
- Autonomous driving: understanding AI decisions in the case of road accidents, for example.
The stakes are so high when it comes to explainable AI and the data used for training that Europe will be adopting a regulatory framework in 2025: the AI Act! Explainability is at the heart of these regulations, which result in the classification of models into 3 levels of risk:
- Unacceptable: These systems are prohibited because they threaten fundamental rights, such as cognitive manipulation, social rating and certain forms of real-time biometric surveillance.
- High: These systems are subject to strict requirements in terms of transparency, robustness and auditability. They concern sensitive areas such as health, education, recruitment or justice.
- Limited and minimal risk: AI with limited risk must simply comply with transparency obligations (e.g. mention that content is generated by AI). AIs with minimal risk (e.g. spam filters) are not subject to any particular requirements.
The European AI Regulation (or AI Act) was published in July 2024 in the Official Journal of the European Union (OJEU) and will gradually come into force from 1 August 2024.
The three key explainability techniques for understanding how AI works :
Explainable AI can be divided into three categories of techniques: global explainability, local explainability and cohort explainability.
- Global explainability: this aims to explain the behaviour of the model as a whole, by identifying the features that influence the model’s overall predictions. This method provides information to stakeholders about the features used by the model when making decisions, as in the case of a recommendation model to understand which features are most engaging for customers.
- Local Explainability: this is used to understand the behaviour of the model at the level of each feature in the data, and to determine how each feature individually contributes to the model’s prediction. This method is particularly useful for identifying the reasons for a problem in production or for discovering the elements that have the greatest influence on the decisions made by the model. This approach is particularly beneficial in sectors such as finance and health, where individual characteristics are very important.
- Cohort explainability: this lies between global and local explainability, and explains how data segments influence the model’s predictions. This method is useful for explaining differences in predictions between different groups of data, as when validating a model, or for understanding outliers and predictions.
Data governance: a major challenge for ensuring the responsible use of AI
Data governance plays a crucial role in ensuring the quality, protection and accountability of data use. It ensures that data is used ethically and responsibly, in compliance with current laws and standards. Controlling data is also important for ensuring trust in the companies that use their data. However, AI algorithms are often criticised for their lack of transparency and accountability, which can have negative consequences for users.
Data governance therefore becomes a major prerequisite for the generation of model training data and its traceability (which source system(s), which transformation(s), completeness, etc.). There is therefore a real need for traceability of the data used during the model training phases.
What are the challenges for more ethical AI?
For more ethical AI, data governance will promote accountability and transparency by working on the following areas:
- Data quality: The data used to train AI models must be of high quality and free from bias. If the data is incomplete, inaccurate or biased, this can affect the accuracy and reliability of the AI model.
- Data confidentiality and security: The data used for AI may contain sensitive personal information. Confidentiality and security must be guaranteed throughout the data life cycle, from collection to destruction. It is possible to go through an anonymisation stage in order to limit the impact.
- Ownership of data: This concept can be complex, particularly in cases where data is collected from third parties or in shared data environments. It is important to clarify data ownership rights to avoid conflicts.
- Governance and regulation: Companies must comply with regulations on the protection of citizens’ and users’ data, such as the RGPD in Europe, the CCPA in California and now the AI Act. They must also put in place data governance policies and procedures to ensure responsible and ethical use of data.
Data governance and explainable AI: an essential duo?
Explainable artificial intelligence and data governance are therefore closely linked. Explainable AI is a methodology that depends heavily on the quality and availability of data.
Data governance plays a key role in providing reliable, high-quality data for AI systems. But (explainable) AI also has a role to play in helping to improve data governance! It can help to identify the characteristics of the data that are most important for the decisions made by AI models.
In conclusion, explainable artificial intelligence and data governance are key areas for ensuring that AI plays a responsible and ethical role in our modern society.
A data platform like Phoenix is an indispensable component of an explainable AI scenario: it provides complete traceability of the data, from its identification in the catalogue to its preparation. It can even expose AI models thanks to its API management module. The result is centralised governance of AI, from its creation to its use.
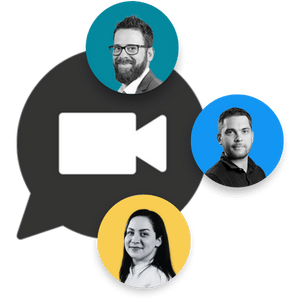
Want to discuss your data management challenges with an expert?